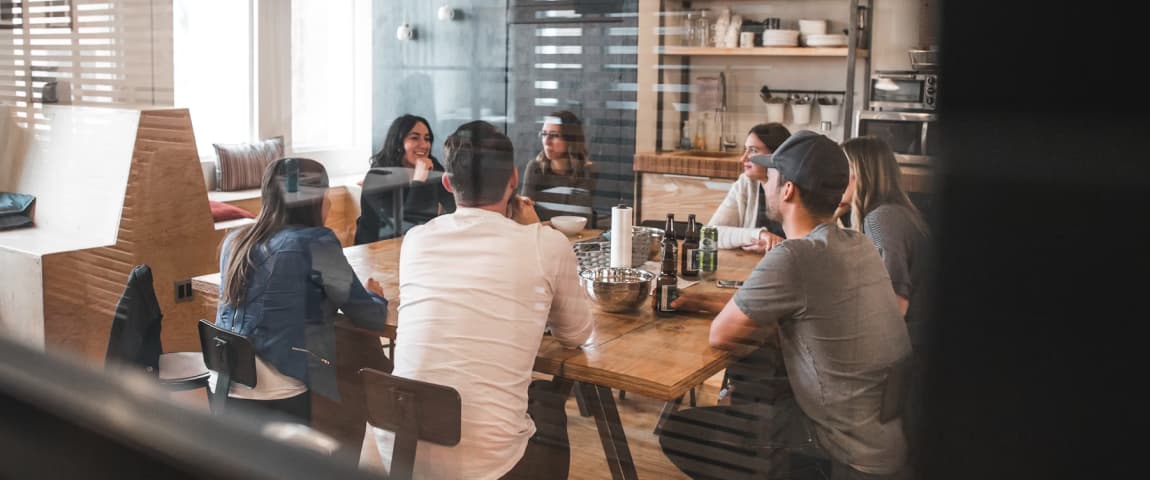
How do I think about it?
One way of thinking about tables in AMC is to try and understand the grain/dimensionality of a row. Once we begin to understand how a row is composed it becomes easier to use the imagination and creativity to aggregate and manipulate the data. In this case, a row represents different types of events. A single row can be an impression from an SP ad or a click event from an SB campaign. In other words, this table is very granular and composed of individual impressions and clicks and all of the associated metrics and dimensions of said events. When you look at the table and see the field prefix ‘event’ you know that this is either an impression or a click event. Immediately we can start working with timestamps, attribution, paths because this data is available to us per user_id. It’s possible to take a single user and map out their entire journey of impressions, clicks, purchase over time. However, in practice this isn’t the case as Amazon Marketing Cloud is a data clean room. Data must be aggregated and adhere to aggregation thresholds so that any one person’s data is not exposed
Important Notes
- ad_product_type is the dimension field that differentiates between each of the sponsored ads types. ad_product_type = sponsored_brands | sponsored_products | sposnored_display.
- Sponsored ads are purchased on a pay-per-click model. So the cost structure will look like SUM(spend) where clicks=1. Spend will have no value for impression rows, in contrast to DSP.
How do I use it?
Almost always in conjunction with other tables. The use cases below are interesting, but I find myself more often than not taking the metrics I need from sponsored_ads_traffic and unioning the data with DSP for further analysis. Most of my work has included a holistic view which requires including DSP. It’s also a virtue of having AMC that you always have DSP that you are utilizing. As AMC becomes more prevalent and accessible to more companies, I think building customer journey analysis and audiences based off of sponsored_ads_traffic tables is valuable. Especially as it also includes keyword level data. This opens up a new dimension of targeting since we can now see users who have searched, purchased, and audiences that searched and did not purchase. Like other tables in AMC, I like to use them to create audiences.
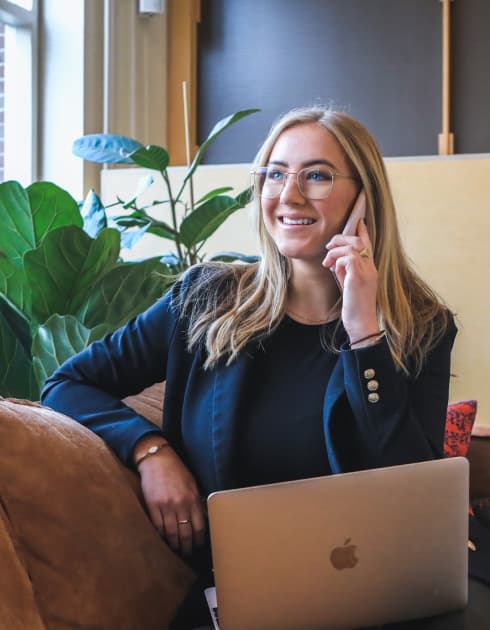
Use Cases
Performance Analysis by Ad Type: By using the ad_product_type field, advertisers can analyze the performance of different ad types (SP, SB, SD) separately. This can help in understanding which ad type is driving more clicks, impressions, and ultimately conversions.
Attribution Modelling: By joining this table with conversion data, advertisers can build custom attribution models to understand the impact of different touchpoints in the conversion funnel. This can help in assigning appropriate credit to each interaction that led to a conversion.
Cost Analysis: Since spend data is tied to click events, advertisers can compute various cost metrics such as Cost-Per-Click (CPC), Return on Ad Spend (ROAS), and Cost-Per-Acquisition (CPA). This helps in ensuring that the advertising spend is optimized and delivering a good return on investment.